Chapter 5 Monte Carlo¶
Monte Carlo Methods are introduced in this chapter.
MC is able to approximate solutions to problems that do not have a full MDP model with all of its action and transition probabilities.
IntroRL provides “Black Box” models that simulate a full MDP models responses. A black box will respond to (State, Action) inputs with (Next State, Reward) responses.
First Visit MC Prediction¶
The figure below is from Sutton & Barto page 92 describing the logic behind First Visit Monte Carlo.
It is used to calculate an estimate of the value function, V(s) for a given policy.
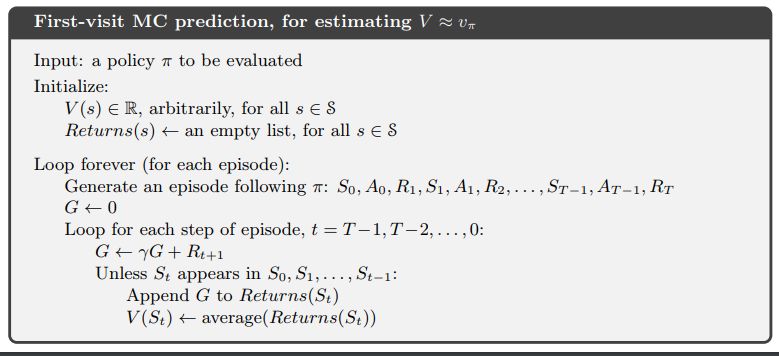
In IntroRL, the main loop of the First Visit MC Prediction is shown below.
In order to assure that all start states are represented, it loops through a randomized, full list of non-terminal states on each of the main loops. An episode is created for each start state using the input policy and the returns are saved to a running-average state value object.
while (num_episodes<=max_num_episodes-1) and keep_looping:
keep_looping = False
abserr = 0.0 # calculated below as part of termination criteria
# policy evaluation
for start_hash in Env.iter_all_action_states( randomize=True ):
# break from inner loop if max_num_episodes is hit.
if num_episodes >= max_num_episodes:
break
make_episode(start_hash, policy, Env, Env.terminal_set, episode=episode,
max_steps=max_episode_steps, eps_greedy=None)
num_episodes += 1
for dr in episode.get_rev_discounted_returns( gamma=gamma,
first_visit=first_visit,
visit_type='S'):
(s_hash, a_desc, reward, sn_hash, G) = dr
state_value_ave.add_val( s_hash, G)
abserr = state_value_ave.get_biggest_action_state_err()
if abserr > max_abserr:
keep_looping = True
if num_episodes < min_num_episodes:
keep_looping = True # must loop for min_num_episodes at least
First Visit Monte Carlo Souce Code
Example 5.1 Blackjack¶
Page 93 of Sutton & Barto uses the game of blackjack to illustrate the use of First Visit MC Prediction.
The IntroRL code below shows the same results as example 5.1.
from introrl.mc_funcs.mc_fv_prediction import mc_first_visit_prediction
from introrl.black_box_sims.blackjack_sim import BlackJackSimulation
from introrl.policy import Policy
from introrl.agent_supt.state_value_run_ave_coll import StateValueRunAveColl
BJ = BlackJackSimulation()
pi = Policy( environment=BJ )
# default policy is hit on everything except 20 & 21.
pi.set_policy_from_piD( BJ.get_default_policy_desc_dict() )
sv = StateValueRunAveColl( BJ )
mc_first_visit_prediction( pi, sv, max_num_episodes=10000, max_abserr=0.001, gamma=1.0)
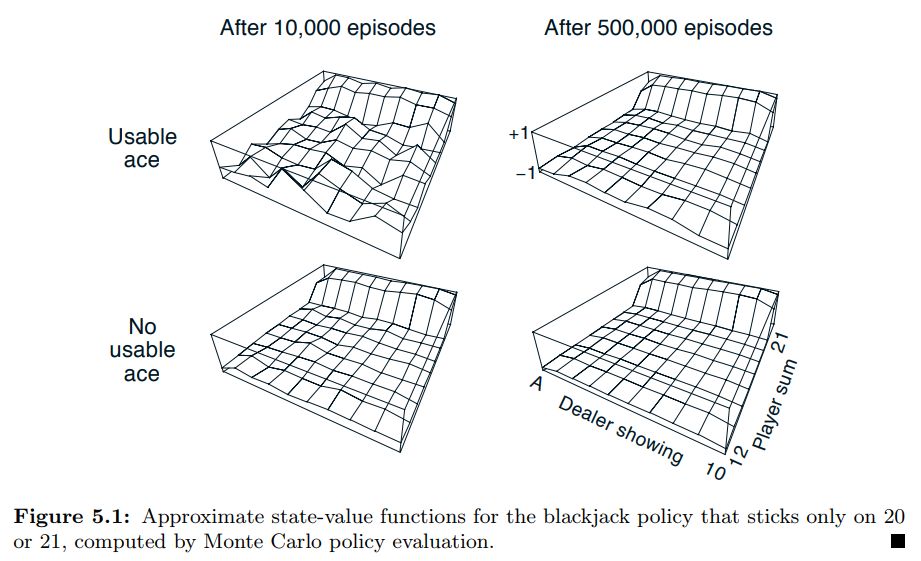
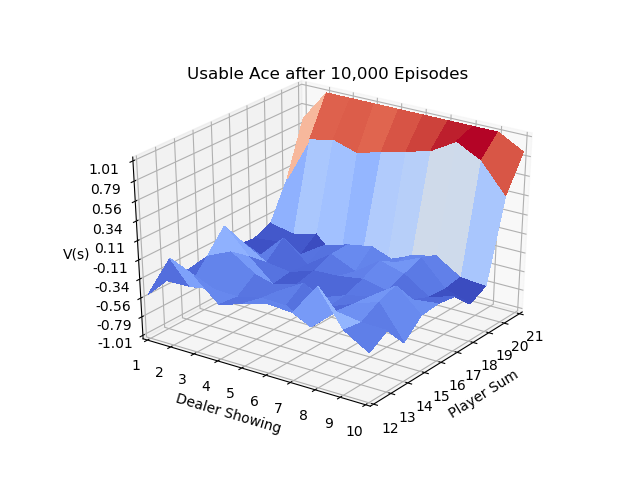
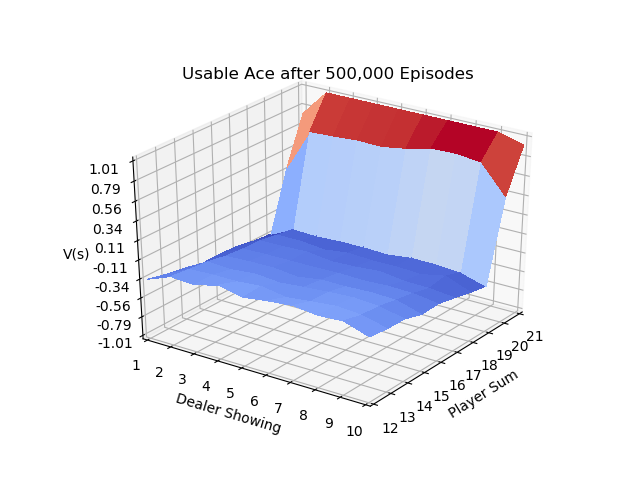
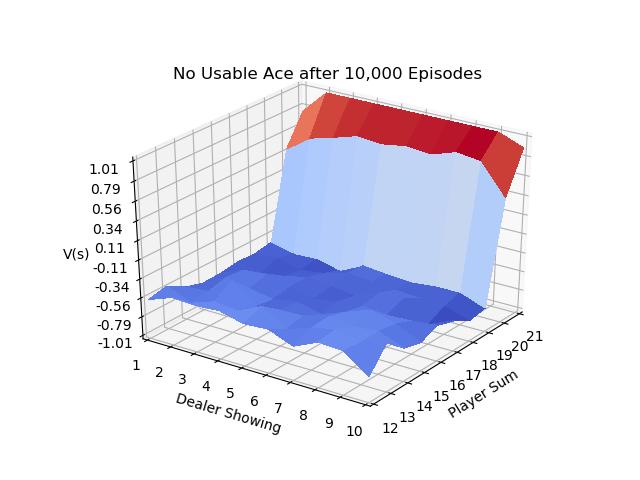
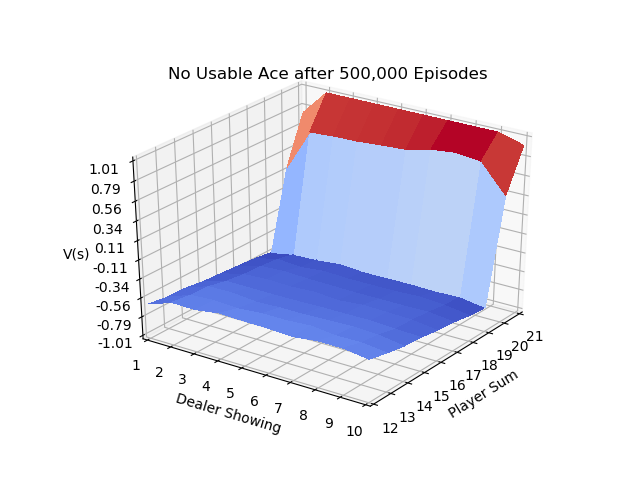
The 3D plot above is created by first running a script to make a data file, Make BJ Data File Code
And then running these scripts to create 3D Plot of 10,000 Episodes Souce Code and 3D Plot of 500,000 Episodes Souce Code
First Visit MC Exploring Starts¶
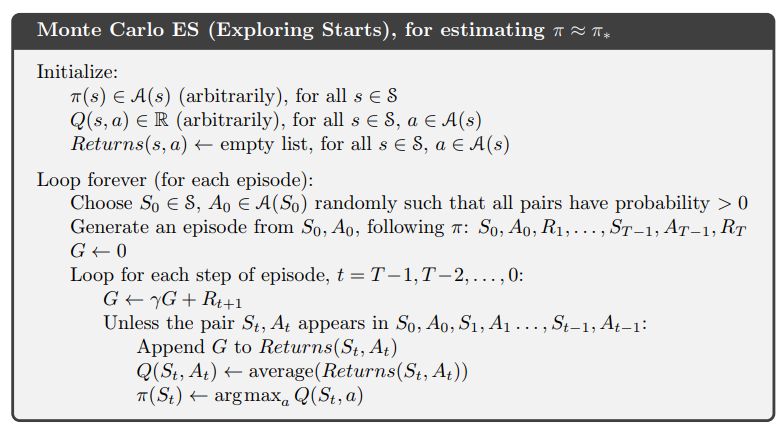
Example 5.3: Solving Blackjack¶
Example 5.3 in Sutton & Barto applies Monte Carlo Exploring Starts to blackjack with the following result.
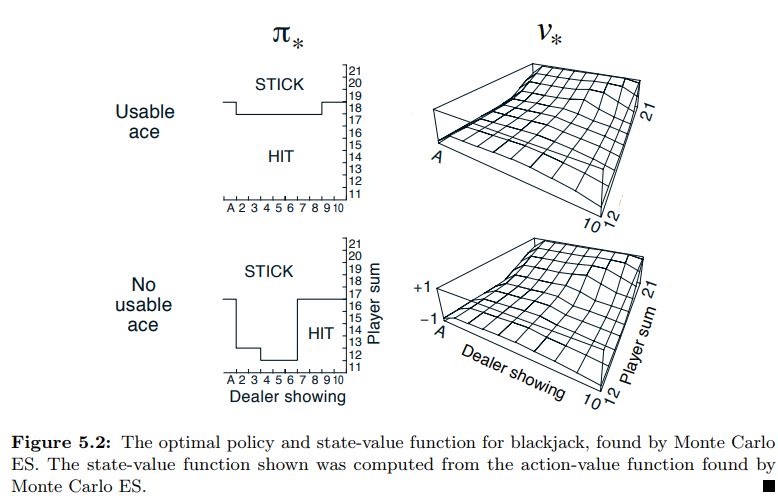
Using the following code from IntroRL, the same policy as Sutton & Barto is obtained.
from introrl.mc_funcs.mc_exploring_starts import mc_exploring_starts
from introrl.black_box_sims.blackjack_sim import BlackJackSimulation
BJ = BlackJackSimulation()
pi, av = mc_exploring_starts( BJ, initial_policy='default',
first_visit=True,
do_summ_print=True, showRunningAve=False, fmt_Q='%g', fmt_R='%g',
max_num_episodes=100000000, min_num_episodes=10,
max_abserr=0.000001, gamma=0.9,
iteration_prints=0)
pi.save_diagram( BJ, inp_colorD=None, save_name='blackjack_policy',
show_arrows=False, scale=0.5, h_over_w=0.8,
show_terminal_labels=False)
Using exploring starts with a very large number of episodes, IntroRL outputs the following results which agree with the answer given on page 100 of Sutton & Barto
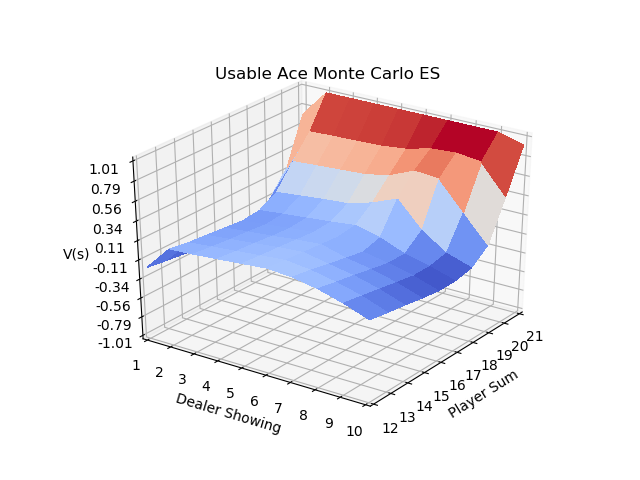
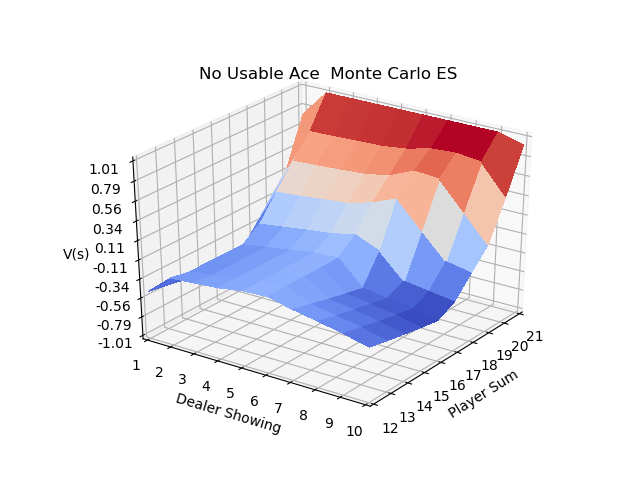
The 3D plot above is created by first running a script to make a data file, Make ES Data File Code
And then running 3D Plot ES Souce Code
The policy is also in agreement.:
___ Policy Summary ___
Nstate-actions=210
___ BlackJack Simulation Policy Summary ___
* * * * * * * * * * | Lose || U
* * * * * * * * * * | Draw || s
* * * * * * * * * * | Win || ab
S S S S S S S S S S | 21 || l
S S S S S S S S S S | 20 || e
S S S S S S S S S S | 19 || A
Hit S S S S S S S Hit Hit | 18 || ce
Hit Hit Hit Hit Hit Hit Hit Hit Hit Hit | 17 ||
Hit Hit Hit Hit Hit Hit Hit Hit Hit Hit | 16 ||
Hit Hit Hit Hit Hit Hit Hit Hit Hit Hit | 15 || P
Hit Hit Hit Hit Hit Hit Hit Hit Hit Hit | 14 || la
Hit Hit Hit Hit Hit Hit Hit Hit Hit Hit | 13 || y
Hit Hit Hit Hit Hit Hit Hit Hit Hit Hit | 12 || er
* * * * * * * * * * | Lose ||
* * * * * * * * * * | Draw || Su
* * * * * * * * * * | Win || m
S S S S S S S S S S | 21 ||
S S S S S S S S S S | 20 ||
S S S S S S S S S S | 19 || N
S S S S S S S S S S | 18 || o
S S S S S S S S S S | 17 || U
Hit S S S S S Hit Hit Hit Hit | 16 || sa
Hit S S S S S Hit Hit Hit Hit | 15 || b
Hit S S S S S Hit Hit Hit Hit | 14 || l
Hit S S S S S Hit Hit Hit Hit | 13 || e
Hit Hit Hit S S S Hit Hit Hit Hit | 12 || A
Hit Hit Hit Hit Hit Hit Hit Hit Hit Hit | 11 || ce
_1___2___3___4___5___6___7___8___9___10__
______________ Dealer Showing _____________
Note that the above solution uses a gamma of 0.9. Interestingly, when run with a gamma of 1.0, the solution is very slightly different for the “No Usable Ace” and player sum of 12:
___ Policy Summary ___
Nstate-actions=210
___ BlackJack Simulation Policy Summary ___
* * * * * * * * * * | Lose || U
* * * * * * * * * * | Draw || s
* * * * * * * * * * | Win || ab
S S S S S S S S S S | 21 || l
S S S S S S S S S S | 20 || e
S S S S S S S S S S | 19 || A
Hit S S S S S S S Hit Hit | 18 || ce
Hit Hit Hit Hit Hit Hit Hit Hit Hit Hit | 17 ||
Hit Hit Hit Hit Hit Hit Hit Hit Hit Hit | 16 ||
Hit Hit Hit Hit Hit Hit Hit Hit Hit Hit | 15 || P
Hit Hit Hit Hit Hit Hit Hit Hit Hit Hit | 14 || la
Hit Hit Hit Hit Hit Hit Hit Hit Hit Hit | 13 || y
Hit Hit Hit Hit Hit Hit Hit Hit Hit Hit | 12 || er
* * * * * * * * * * | Lose ||
* * * * * * * * * * | Draw || Su
* * * * * * * * * * | Win || m
S S S S S S S S S S | 21 ||
S S S S S S S S S S | 20 ||
S S S S S S S S S S | 19 || N
S S S S S S S S S S | 18 || o
S S S S S S S S S S | 17 || U
Hit S S S S S Hit Hit Hit Hit | 16 || s
Hit S S S S S Hit Hit Hit Hit | 15 || ab
Hit S S S S S Hit Hit Hit Hit | 14 || l
Hit S S S S S Hit Hit Hit Hit | 13 || e
Hit Hit Hit Hit S S Hit Hit Hit Hit | 12 || A
Hit Hit Hit Hit Hit Hit Hit Hit Hit Hit | 11 || ce
_1___2___3___4___5___6___7___8___9___10__
______________ Dealer Showing _____________
Also of note, the answer from Shangtong Zhang results in a 3rd answer for the “No Usable Ace” and player sum of 12.
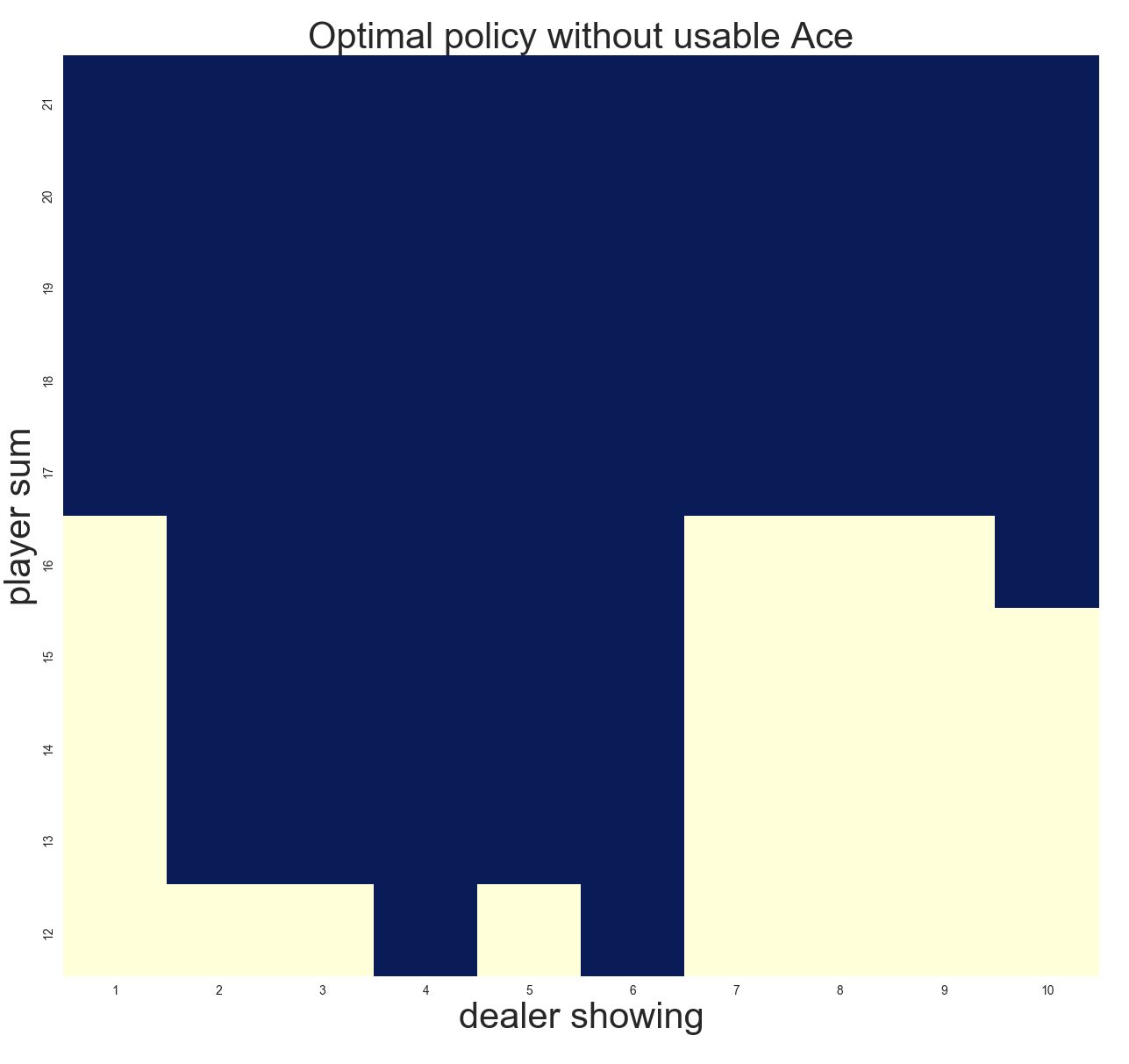
First Visit Epsilon-Greedy MC¶
The algorithm for an epsilon-greedy policy is given on page 101 of Sutton & Barto
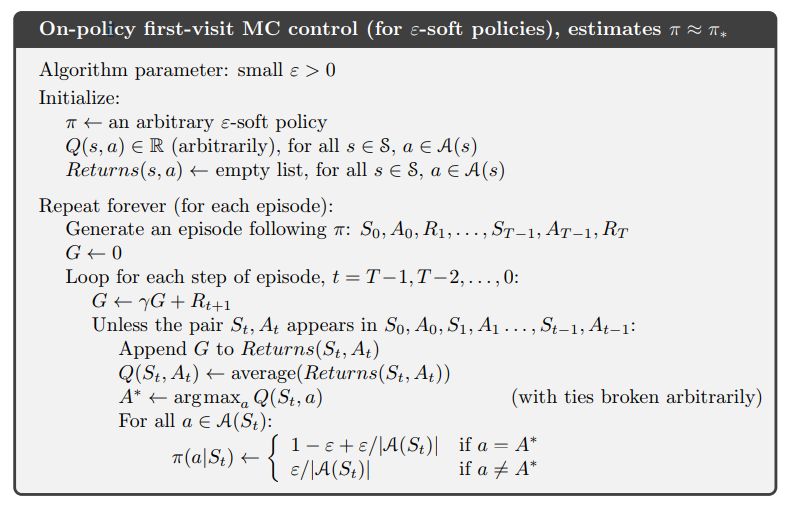
The main loop of the implementation of epsilon-greedy Monte Carlo in IntroRL is shown below.
limited_start_stateL = environment.limited_start_state_list()
while (num_episodes<=max_num_episodes-1) and keep_looping :
keep_looping = False
abserr = 0.0 # calculated below as part of termination criteria
Nterminal_episodes = set()
# Iterate over a list of known possible start states
if use_list_of_start_states:
loop_stateL = limited_start_stateL
random.shuffle( loop_stateL )
else:
#loop_stateL = [ random.choice( limited_start_stateL ) ]
loop_stateL = [ environment.start_state_hash ]
for start_hash in loop_stateL:
if iter_all_start_actions:# Iterate over ALL ACTIONS of start_hash
a_descL = environment.get_state_legal_action_list( start_hash )
else:
a_desc = policy.get_single_action( start_hash )
# if not iterating all actions, make sure first action has eps-greedy applied
a_desc = eps_greedy( a_desc,
environment.get_state_legal_action_list( start_hash ) )
a_descL = [ a_desc ]
# randomize action order
random.shuffle( a_descL )
for a_desc in a_descL:
# break from inner loop if max_num_episodes is hit.
if num_episodes >= max_num_episodes:
break
make_episode(start_hash, policy,
environment, environment.terminal_set,
episode=episode, first_a_desc=a_desc,
max_steps=max_episode_steps, eps_greedy=eps_greedy)
eps_greedy.inc_N_episodes()
num_episodes += 1
if episode.is_done():
Nterminal_episodes.add( start_hash )
for dr in episode.get_rev_discounted_returns( gamma=gamma,
first_visit=first_visit,
visit_type='SA'):
# look at each step from episode and calc average Q(s,a)
(s, a, r, sn, G) = dr
action_value_ave.add_val( s, a, G)
aL = environment.get_state_legal_action_list( s )
if aL:
best_a_desc, best_a_val = aL[0], float('-inf')
bestL = [best_a_desc]
for a in aL:
q = action_value_ave.get_ave( s, a )
if q > best_a_val:
best_a_desc, best_a_val = a, q
bestL = [ a ]
elif q == best_a_val:
bestL.append( a )
best_a_desc = random.choice( bestL )
policy.set_sole_action(s, best_a_desc)
abserr = action_value_ave.get_biggest_action_state_err()
if abserr > max_abserr:
keep_looping = True
if num_episodes < min_num_episodes:
keep_looping = True # must loop for min_num_episodes at least
First Visit Epsilon-Greedy MC Souce Code
Invoking the above code with the IntroRL code below, gives the correct policy and state values.
from introrl.mc_funcs.mc_fv_epsilon_greedy import mc_epsilon_greedy
from introrl.black_box_sims.blackjack_sim import BlackJackSimulation
BJ = BlackJackSimulation()
pi, av = mc_epsilon_greedy( BJ, initial_policy='default',
read_pickle_file='blackjack_epsgreedy',
save_pickle_file='blackjack_epsgreedy',
first_visit=True,
do_summ_print=True, showRunningAve=False, fmt_Q='%g', fmt_R='%g',
show_initial_policy=True,
max_num_episodes=5000000, min_num_episodes=10,
max_abserr=0.0001, gamma=0.9,
iteration_prints=0,
max_episode_steps=10000,
epsilon=0.1, const_epsilon=True)
The policy from the above code is:
___ Policy Summary ___
Nstate-actions=210
___ BlackJack Simulation Policy Summary ___
* * * * * * * * * * | Lose || U
* * * * * * * * * * | Draw || s
* * * * * * * * * * | Win || ab
S S S S S S S S S S | 21 || l
S S S S S S S S S S | 20 || e
S S S S S S S S S S | 19 || A
Hit S S S S S S S Hit Hit | 18 || ce
Hit Hit Hit Hit Hit Hit Hit Hit Hit Hit | 17 ||
Hit Hit Hit Hit Hit Hit Hit Hit Hit Hit | 16 ||
Hit Hit Hit Hit Hit Hit Hit Hit Hit Hit | 15 || P
Hit Hit Hit Hit Hit Hit Hit Hit Hit Hit | 14 || la
Hit Hit Hit Hit Hit Hit Hit Hit Hit Hit | 13 || y
Hit Hit Hit Hit Hit Hit Hit Hit Hit Hit | 12 || er
* * * * * * * * * * | Lose ||
* * * * * * * * * * | Draw || Su
* * * * * * * * * * | Win || m
S S S S S S S S S S | 21 ||
S S S S S S S S S S | 20 ||
S S S S S S S S S S | 19 || N
S S S S S S S S S S | 18 || o
S S S S S S S S S S | 17 || U
Hit S S S S S Hit Hit Hit S | 16 || s
Hit S S S S S Hit Hit Hit Hit | 15 || ab
Hit S S S S S Hit Hit Hit Hit | 14 || l
Hit S S S S S Hit Hit Hit Hit | 13 || e
Hit Hit Hit S S S Hit Hit Hit Hit | 12 || A
Hit Hit Hit Hit Hit Hit Hit Hit Hit Hit | 11 || ce
_1___2___3___4___5___6___7___8___9___10__
______________ Dealer Showing _____________